Churn modelling for targeted marketing
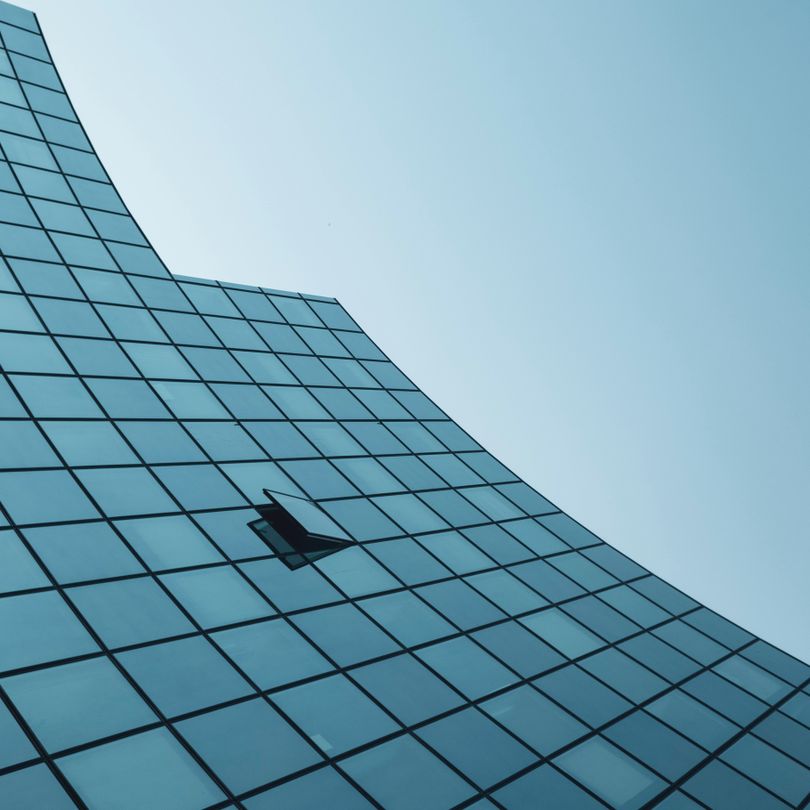
Problem - A client in the energy industry needs to identify among their business customers who are more likely to terminate their contracts in the coming month. The client will offer right marketing and promotion campaigns to their most likely churn customers.
Approach - Utilize ML models to score the churn likelihood for all the customers monthly, based on the updated customer data
Results - The solution automatically identifies 95% of churnable customers with a precision of ~80%. The client can offer campaigns to the right targeted customers, saving costs and increase the customer retention.
Data - Transactions, customer service, background, competitor pricing, temperature
Tools - Database: SQL - Data science: Python, Scikit-learn, Jupyter, ML Flow, AWS Sagemaker - Visualization: Tableau